Vol. 17, n. 3, novembre 2024
STRUMENTI
Technostress legato alla Formazione a Distanza
Un Contributo alla Validazione della Italian Technostress Scale for University Students in Technology-enhanced learning
Giovanni Schettino e Vincenza Capone1
Sommario
L’adozione dell’apprendimento potenziato dalla tecnologia (TEL) costituisce un fenomeno ampiamente diffuso nelle organizzazioni accademiche, principalmente nella forma dell’apprendimento a distanza. Sebbene siano noti i suoi vantaggi sia a livello individuale che organizzativo, le richieste associate a tale contesto di apprendimento possono spingere gli studenti a soffrire di uno stress correlato alla tecnologia o technostress. Spinti dalla necessità di misurare adeguatamente il technostress negli studenti cinesi, Wang e colleghi hanno validato una scala per questa specifica popolazione. Il presente studio mira a sviluppare una validazione iniziale della versione italiana (IT-TSUS) di tale strumento esaminando le proprietà psicometriche in un campione di 550 studenti universitari iscritti a un corso di laurea erogato online. Le analisi fattoriali confermative hanno confermato la struttura fattoriale unidimensionale in linea con la validazione originale della scala. Inoltre, i risultati hanno riportato una buona coerenza interna della scala e la sua validità esterna. Alla luce di quanto evidenziato, il presente studio fornisce evidenze a supporto delle buone proprietà psicometriche dell’IT-TSUS e ne suggerisce la sua appropriatezza nell’utilizzo nell’ambito della ricerca e dell’intervento all’interno del contesto italiano.
Parole chiave
Technostress, TEL, Studenti universitari, Proprietà psicometriche, Validazione scala.
INSTRUMENTS
Technostress Related to Distance Education
A Contribution to the Validation of the Italian Technostress Scale for University Students in Technology-Enhanced Learning
Giovanni Schettino and Vincenza Capone2
Abstract
The adoption of technology-enhanced learning (TEL) is widespread in academic organizations, mainly in the form of distance learning. While its advantages are well acknowledged at the individual and organizational levels, the demands associated with such a learning context can lead students to suffer from technology-related stress or technostress. To address the need for proper measurement of technostress in Chinese students, Wang and colleagues validated a scale specifically for this population. The present study aimed to develop an initial Italian validation (IT-TSUS) of such an instrument by examining its psychometric properties in a sample of 550 university students enrolled in a degree course delivered online. Confirmatory factor analyses confirmed the one-dimensional factor structure consistent with the original validation. In addition, the findings reported a good internal consistency of the scale and its external validity. In light of the above, the present study provides evidence supporting the good psychometric properties of the IT-TSUS and suggests its suitability for use in research and intervention within the Italian context.
Keywords
Technostress, TEL, University students, Psychometric properties, Scale validation.
Introduction
The growing implementation of technology-enhanced learning (TEL) in higher education has significantly reshaped the learning environment, mainly through distance education (Ferraro et al., 2020; Soffer et al., 2010).
Such a phenomenon has provided students with unprecedented opportunities and challenges. Indeed, while technology offers numerous advantages — both for students and educational organizations (Adedoyin & Soykan, 2023) — it also can increase the risk for negative psychosocial outcomes related to technology-related stress. Specifically, literature in this field has shown a growing concern for technostress, a modern stressor arising from interactions with technology and potentially resulting in decreased well-being (Asad et al., 2023), poorer academic performance (Qi, 2019; Sethi et al., 2021), and higher prevalence of anxious and depressive symptoms (Torales et al., 2022) among students.
Grounded in Brod’s (1982) initial conceptualization of such a phenomenon among workers, technostress reflects the adverse psychological impacts resulting from factors such as information overload, the complexities of new technologies, and the erosion of boundaries between work and personal life (Tarafdar et al., 2011). The person-environment fit model can support understanding technostress in university students (P-E fit; Edwards et al., 1998). This model not only identifies the potential stressors but also offers a framework for addressing them. It posits that stress can be determined by a misalignment between individuals’ needs and capabilities and the demands and resources of their environment. In the context of distance learning, students’ technological skills, adaptability, and learning styles represent personal factors. The environment-related factors include the specific technologies adopted by the university, the level of support provided, and the overall digital culture fostered by the organization. When there is a mismatch between these individual and environmental factors, students may experience heightened technostress (Erdogan et al., 2022).
Additionally, it is worth noting that technostress and its consequences vary depending on the specific technology involved. In other words, different types of technology — learning management systems, virtual classrooms, or collaboration tools — require different cognitive and emotional demands on users, which can influence the manifestation of technostress. This variability, in turn, underscores the necessity for effective instruments that measure technostress not only generally but also in relation to the specific technology and population. Without technology-sensitive measurement tools, interventions may lack effectiveness, failing to address the root causes of such stress for different groups of users. Following this reasoning, Wang and colleagues (2020) validated an agile technostress measure (The P-E fit scale of technostress for university students in technology-enhanced learning) to assess such stress among Chinese students. Specifically, the authors developed an initial technostress scale consisting of 13 items and reflecting the core components of the P-E fit theory. Through three rounds of study, they validated a final version of the technostress scale, identifying a one-factor structure and demonstrating robust psychometric properties. This latter version includes eight items designed to measure university students’ technostress in TEL.
Validation processes require a series of steps and procedures to guarantee the reliability of the instrument in question. The role of the administration context is of paramount importance in this regard. Consequently, this paper aims to present a validation procedure for the techno-stress scale designed for use with Italian students (Schettino et al., 2024). More in detail, the present study is a second validation step of the Italian adaptation of the tool based on the Wang et al. (2020) scale. In such a way, the instrument could provide valuable data to develop effective interventions in Italian educational organizations, thereby improving student well-being and fostering a more supportive learning environment.
Methods
Participants
A convenience sample of 550 Italian students (84% female) was recruited for the study. Participants of the study were attending a degree course in psychology delivered online. Most were women (84%) and were unemployed (86.4%). The mean age of participants was 20.69 (SD = 1.99; range = 18-33 years of age). Among the respondents, 44% were attending the first year of the course, while 56% were attending the last year.
Procedures
Participants were informed about the anonymity of data collection and signed the informed consent form in accordance with EU Regulation 679/2016. They then completed an online self-reported questionnaire on the Google Forms platform. The questionnaire took approximately 20 minutes to complete, and each item required a mandatory answer; hence, no respondents reported missing values. Research participation was voluntary, and participants received no reward. Data was collected in March 2021.
Measures
In the first section of the questionnaire, participants filled out the informed consent form. Then, they were asked for their demographic information (e.g., age and work conditions). In addition, the following measures were administered to all the participants in the same order.
The Italian Technostress Scale for University Students in Technology-Enhanced Learning (IT-TSUS): the tool consists of 8 closed questions (e.g., «I feel stressed to adapt to technology-enhanced learning») assessing university students’ technostress. Items were rated on a 5-point Likert scale (0 = strongly disagree; 4 = strongly agree). The original version of the scale (Wang et al., 2020) was translated from English into Italian using a parallel back-translation procedure. A bilingual individual provided an Italian translation of the scale, and a second bilingual individual translated this version back into English. According to the translators, the original and back-translated English versions did not differ significantly. Lastly, feedback from six university students contributed to enhancing the wording and clarity of the items. In this study, Cronbach’s alpha of the IT-TSUS was .92. See Table A1 in Appendix 1 for the complete set of items with means and standard deviation.
The Technostress Creators Scale (TCS; Molino et al., 2020; Ragu-Nathan et al., 2008) is an instrument that measures workers’ technostress. The tool consists of 11 items («I am forced by technology to work much faster») evaluated on a 5-point Likert scale from strongly disagree (1) to strongly agree (5). Cronbach’s alpha for The Technostress Creators Scale was .83.
The Italian Mental Health Continuum Short Form (MHC-SF; Petrillo et al., 2015) consists of 14 statements assessing psychosocial well-being (e.g., «During the past month, how often did you feel that you had experiences that challenged you to grow and become a better person»). Respondents express how much they functioned in a specific manner by adopting a graduated scale from 0 (none of the time) to 5 (all of the time). MHC-SF reported a Cronbach’s alpha of .90.
Data Analyses
Data were subjected to confirmatory factor analysis (CFA) with maximum likelihood estimation method by using R in order to test the one-dimensional factor structure, in line with the original validation study (Wang et al., 2020). To evaluate the solution, we took into account the goodness of different fit indexes (Hu & Bentler, 1999). Specifically, a normed model chi-square (χ2/df) value of < 3.00 indicates a good fit. We also considered adequate comparative fit index (CFI) and the Tucker–Lewis index (TLI) values above .90 (Bentler, 2006; Byrne et al., 1989), root-mean-square error of approximation (RMSEA) values below or equal to .06, and root-mean-square residual (RMSR) values equal to or below .09 (Hu & Bentler, 1999). Moreover, to test internal consistency, the corrected correlations between the score of the items and the IT-TSUS, as well as Cronbach’s alpha, were computed. Finally, the external validity of the scale was evaluated by performing a correlational analysis.
Results
CFA results showed a poor overall fit (χ2(20) = 149.179, p < .001, χ2/df = 7.46; CFI = .94, TLI = .91, SRMR = .12, RMSEA = .04). Modification indices suggested adding the error covariance between items 6 and 7, items 5 and 6, items 4 and 7, items 4 and 8, and items 5 and 8. After adding these constraints, an acceptable fit for the one-factor solution was obtained (χ2(15) = 59.893, p < .001, χ2/df = 3.99, CFI = .98, TLI = .96, SRMR = .03, RMSEA = .08) (Figure 1). Concerning internal consistency, a good Cronbach’s α coefficient emerged, namely .92.
Figure 1
Path diagram from the CFA of the IT-TSUS
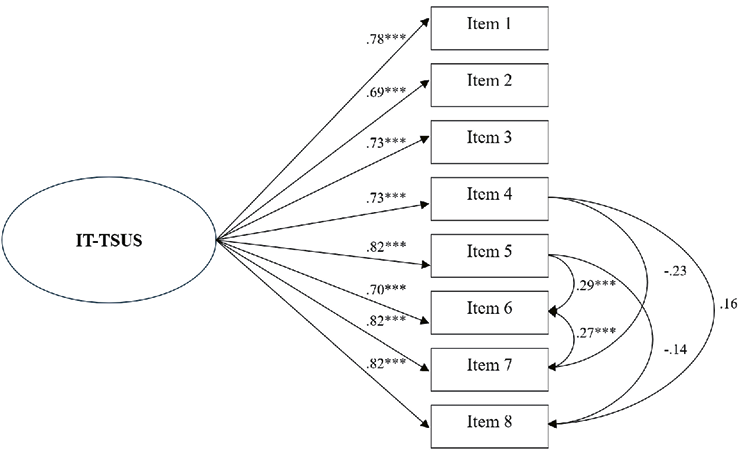
Note. ***p < .001.
Finally, correlational analyses (Table 1) showed that associations between the IT-TSUS and other scales were statistically significant (p < .01). More in detail, the IT-TSUS was positively associated with TCS (r = .66) and negatively with psychosocial well-being, which was evaluated through MHC-SF (r = -.25).
Table 1
Descriptive statistics and Pearson’s correlations among the variables
Mean (SD) |
Range |
1. |
2. |
3. |
|
Technostress (IT-TSUS) |
1.97 (.89) |
0-4 |
1 |
||
Technostress (TCS) |
2.73 (.64) |
1-5 |
.66** |
1 |
|
Well-being (MHC-SF) |
3.37 (.88) |
0-5 |
- .25** |
- .22** |
1 |
Note. ** p < .01; SD = standard deviation.
Discussion
This study aimed to provide a contribution to the adaptation and validation of the technostress scale developed by Wang et al. (2020) for its adoption in the Italian context. In order to achieve this objective, the factorial structure, internal consistency, and external validity of the scale were evaluated. Our results were satisfactory, demonstrating robust psychometric properties of the IT-TSUS similar to those of the original Chinese version. Specifically, confirmatory factor analysis supported the one-factor structure of the scale. Furthermore, the analyses indicated excellent internal consistency and provided evidence for the external validity of the scale. Notably, positive correlations were found between the IT-TSUS and TCS scales, indicating the scale convergent validity. In contrast, a negative correlation was observed between the IT-TSUS and MHC-SF scales, suggesting that higher levels of technostress are associated with lower levels of mental health and, thereby, the need to address technostress in educational settings.
Despite these results, it is necessary to emphasize some limitations that should be considered in subsequent studies. More in detail, this contribution to the validation of the IT-TSUS scale was conducted on a sample of Italian university students with specific characteristics. Specifically, they were enrolled in the first or final year of a psychology degree programme. Consequently, it is plausible to suppose these students are in a critical phase of career development, a time typically characterized by heightened stress levels due to the uncertainties and challenges associated with career exploration and decision-making (Barbayannis et al., 2022; Bewick et al., 2010). Thus, the inherent stress associated with this career stage may have affected their responses to the technostress scale and the reported levels of technology-related stress. Therefore, further research is needed to examine the psychometric properties of the IT-TSUS scale across different student populations and career stages in order to determine the generalizability of the findings.
In conclusion, these results suggest that the scale can be regarded as a suitable and agile instrument for assessing technostress in the Italian context. Hence, it can be adopted to promptly identify students at higher risk of developing technostress and, thereby, the negative consequences related to this modern disease. In addition, the tool could support professionals within academic organizations by providing them with reliable data to design effective online courses and implement organizational processes to enhance students’ resources in dealing with such a kind of learning context.
References
Adedoyin, O. B., & Soykan, E. (2023). Covid-19 pandemic and online learning: The challenges and opportunities. Interactive Learning Environments, 31(2), 863-875. https://doi.org/10.1080/10494820.2020.1813180
Asad, M. M., Erum, D., Churi, P., & Moreno Guerrero, A. J. (2023). Effect of technostress on Psychological well-being of post-graduate students: A perspective and correlational study of Higher Education Management. International Journal of Information Management Data Insights, 3(1), 100149. https://doi.org/10.1016/j.jjimei.2022.100149
Barbayannis, G., Bandari, M., Zheng, X., Baquerizo, H., Pecor, K. W., & Ming, X. (2022). Academic stress and mental well-being in college students: Correlations, affected groups, and COVID-19. Frontiers in Psychology, 13. https://doi.org/10.3389/fpsyg.2022.886344
Bentler, P. M. (2006). EQS 6 Structural equations program manual. Multivariate Software.
Bewick, B., Koutsopoulou, G., Miles, J., Slaa, E., & Barkham, M. (2010). Changes in undergraduate students’ psychological well-being as they progress through university. Studies in Higher Education, 35(6), 633-645. https://doi.org/10.1080/03075070903216643
Brod, C. (1982). Managing technostress: Optimizing the use of computer technology. Personnel Journal, 61(10), 753–757.
Byrne, B. M., Shavelson, R., & Muthen, B. (1989) Testing for the equivalence of factor covariance and mean structures: The issue of partial measurement invariance. Psychological Bulletin, 105, 456-466. https://doi.org/10.1037/0033-2909.105.3.456
Edwards, J. R., Caplan, R. D., & Harrison, R. V. (1998). Person-environment fit theory: Conceptual foundations, empirical evidence, and directions for future research. In C. L. Cooper (Ed.), Theories of Organizational Stress (pp. 28-67), Oxford University Press.
Erdogan, A., Öztürk, M., Erdogan, P., Zor, R. K., Çinaroglu, S., Öztorun, K., & Kayabas, Ü. (2022). Technostress in medical students during pandemic-prompted distance education: Adaptation of technostress scale based on person-environment misfit theory. Turkish Online Journal of Educational Technology, 21(3), 63-74.
Ferraro, F. V., Ambra, F. I., Aruta, L., & Iavarone, M. L. (2020). Distance learning in the COVID-19 Era: Perceptions in Southern Italy. Education Sciences, 10(12), 355. https://doi.org/10.3390/educsci10120355
Hu, L., & Bentler, P. M. (1999). Cutoff criteria for fit indexes in covariance structure analysis: Conventional criteria versus new alternatives. Structural Equation Modeling: A Multidisciplinary Journal, 6(1), 1-55. https://doi.org/10.1080/10705519909540118
Molino, M., Ingusci, E., Signore, F., Manuti, A., Giancaspro, M. L., Russo, V., Zito, M., & Cortese, C. G. (2020). Wellbeing costs of technology use during Covid-19 remote Wworking: An investigation using the Italian translation of the Technostress Creators Scale. Sustainability, 12(15), 5911. https://doi.org/10.3390/su12155911
Petrillo, G., Capone, V., Caso, D., & Keyes, C. L. M. (2015). The Mental Health Continuum–Short Form (MHC-SF) as a measure of well-being in the Italian context. Social Indicators Research, 121(1), 291-312. https://doi.org/10.1007/s11205-014-0629-3
Qi, C. (2019). A double-edged sword? Exploring the impact of students’ academic usage of mobile devices on technostress and academic performance. Behaviour & Information Technology, 38(12), 1337-1354. https://doi.org/10.1080/0144929X.2019.1585476
Ragu-Nathan, T. S., Tarafdar, M., Ragu-Nathan, B. S., & Tu, Q. (2008). The consequences of technostress for end users in organizations: Conceptual development and empirical validation. Information Systems Research, 19(4), 417-433. https://doi.org/10.1287/isre.1070.0165
Schettino, G., & Capone, V. (2022). Learning design strategies in MOOCs for physicians’ training: a scoping review. International Journal of Environmental Research and Public Health, 19(21), 14247. https://doi.org/10.3390/ijerph192114247
Schettino, G., Marino, L., & Capone, V. (2024). Technology-enhanced learning and well-being: a contribution to the validation of a measure to assess university students’ technostress in the Italian context. International Journal of Mental Health and Addiction, 22(3), 1515-1529. https://doi.org/10.1007/s11469-022-00940-9
Sethi, D., Pereira, V., & Arya, V. (2021). Effect of technostress on academic productivity: E-engagement through persuasive communication. Journal of Global Information Management, 30(5), 1-19.
Soffer, T., Nachmias, R., & Ram, J. (2010). Diffusion of web supported instruction in higher education — The case of Tel-Aviv University. Journal of Educational Technology & Society, 13(3), 212-223.
Tarafdar, M., Tu, Q., Ragu-Nathan, T. S., & Ragu-Nathan, B. S. (2011). Crossing to the dark side: Examining creators, outcomes, and inhibitors of technostress. Communications of the ACM, 54(9), 113-120. https://doi.org/10.1145/1995376.1995403
Torales, J., Torres-Romero, A. D., Di Giuseppe, M. F., Rolón-Méndez, E. R., Martínez-López, P. L., Heinichen-Mansfeld, K. V., Barrios, I., O’Higgins, M., Almirón-Santacruz, J., Melgarejo, O., Ruiz Díaz, N., Castaldelli-Maia, J. M., & Ventriglio, A. (2022). Technostress, anxiety, and depression among university students: A report from Paraguay. International Journal of Social Psychiatry, 68(5), 1063-1070. https://doi.org/10.1177/00207640221099416
Wang, X., Tan, S. C., & Li, L. (2020). Measuring university students’ technostress in technology-enhanced learning: Scale development and validation. Australasian Journal of Educational Technology, 36(4), 96-112. https://doi.org/10.14742/ajet.5329
Appendix 1
Table A1
Items and descriptive analyses of the IT-TSUS
Mean |
SD |
α if deleted |
Item-total corrected correlation |
||
---|---|---|---|---|---|
Item 1 |
Adattarmi alla didattica a distanza mi fa sentire sotto pressione [I feel stressed to adapt to technology-enhanced learning] |
2.05 |
1.13 |
.91 |
.75 |
Item 2 |
Ho difficoltà a seguire in maniera efficace la didattica a distanza perché ho poco tempo e risorse [I find it difficult to effectively use technology-enhanced learning due to my limited investment of time and effort] |
1.74 |
1.09 |
.91 |
.66 |
Item 3 |
Mi sento stressato/a dalle elevate richieste della didattica a distanza alle quali non riesco a far fronte con le mie attuali capacità [I feel stressed to cope with the high demands of technology-enhanced learning with my current capability] |
1.90 |
1.09 |
.91 |
.70 |
Item 4 |
Mi vedo costretto/a cambiare le mie abitudini e scelte di apprendimento per adeguarmi alle richieste della didattica a distanza [I am pressured to change my current learning habit and preference to meet the requirements of technology-enhanced learning] |
2.38 |
1.11 |
.91 |
.69 |
Item 5 |
La didattica a distanza invade diffusamente ogni aspetto della mia formazione, creandomi disagio [I am not comfortable with the pervasive invasion of technology-enhanced learning in all aspects of my study] |
1.74 |
1.08 |
.90 |
.79 |
Item 6 |
Sono infastidito/a dalle varie forme della didattica a distanza [I am irritated by the vast variety of technology-enhanced learning] |
1.80 |
1.12 |
.91 |
.70 |
Item 7 |
Mi stressano le varie forme di didattica a distanza, perché rendono più difficile il mio studio [I feel stressed as the various forms of technology-enhanced learning complicate my study] |
1.98 |
1.16 |
.91 |
.77 |
Item 8 |
Mi sento stressato/a perché la diffusione della didattica a distanza ha portato disordine nelle mie abitudini di studio [I feel stressed as the heavy reliance on technology-enhanced learning in my school disrupts my normal study pattern] |
2.15 |
1.17 |
.90 |
.78 |
Note. Original English items in brackets.
Vol. 17, Issue 3, November 2024